The business world is being revolutionized by artificial intelligence (AI), which is boosting productivity and creating new revenue streams.
Nonetheless, the global market for artificial intelligence (AI) is projected to reach $190 billion by the year 2024, with a compound annual growth rate (CAGR) of 38.1% from 2019 to 2024 as cited by MarketsandMarkets. This groundbreaking technology enables businesses to streamline operations, make data-driven decisions, and offer personalized customer experiences.
On top of it, AI systems can perform tasks that usually require human intelligence, such as prediction, object recognition, speech interpretation, and natural language generation. These systems learn by analyzing vast amounts of data, identifying patterns, and applying these patterns to decision-making. AI’s ability to continuously learn and adapt makes it an essential tool for businesses aiming to stay competitive in a rapidly evolving market.
Consider AI-powered predictive analytics helping businesses anticipate market trends and stay ahead of the competition. Alternatively, AI-driven chatbots enhance customer service by delivering prompt and accurate responses, thereby reducing operating costs and boosting customer satisfaction. AI can also optimize supply chains, personalize marketing strategies, and predict equipment failures before they happen. The possibilities are endless, and the benefits are substantial.
Are you intrigued about how AI can transform your business operations? Let’s explore the incredible opportunities AI offers and how it can redefine success for your business.
The Timeline of Artificial Intelligence – From 1943 to 2024
John McCarthy, known as the father of Artificial Intelligence, first introduced the term "Artificial Intelligence" in 1956. Yet, the journey of AI began in the 1940s. Today, AI is deeply embedded in our daily lives and business practices.
A recent study by Deloitte indicates that 94% of executives view AI as crucial for future success, underscoring its high return on investment. AI's presence is ubiquitous, from smartwatches and personalized recommendations on platforms such as- YouTube and Netflix, to smart home systems, voice assistants, and automated customer support.
In this discussion, we'll explore the evolution of AI, tracing its progress from the 1940s to the 2020s. We'll highlight key milestones and how these advancements have transformed AI from a theoretical concept into a pivotal technology shaping various aspects of our world.
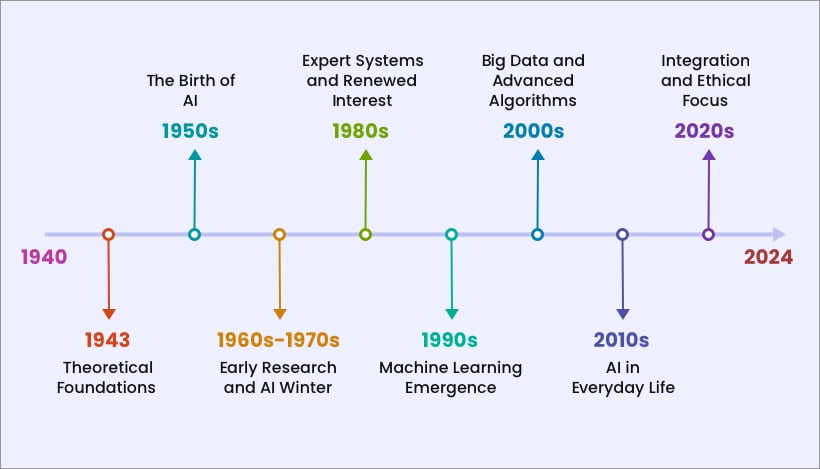
1943: Theoretical Foundations
- McCulloch and Pitts Model: In 1943, Warren McCulloch and Walter Pitts introduced a groundbreaking model of artificial neurons. This model was crucial in laying the groundwork for neural networks, mimicking the functioning of biological neurons. Their work provided a theoretical foundation that would later become essential in the development of artificial intelligence.
1950s: The Birth of AI
- 1950: Alan Turing published his seminal paper "Computing Machinery and Intelligence," where he proposed the Turing Test. This test was designed to evaluate a machine's ability to exhibit intelligent behavior indistinguishable from a human's, marking a significant conceptual advancement in the field of AI.
- 1956: The Dartmouth Conference, organized by John McCarthy, Marvin Minsky, Nathaniel Rochester, and Claude Shannon, officially coined the term "Artificial Intelligence." This event is considered the birth of AI as an academic discipline, setting the stage for future research and development.
1960s-1970s: Early Research and AI Winter
- 1966: Joseph Weizenbaum created ELIZA, one of the earliest natural language processing programs. ELIZA could simulate a conversation with a psychotherapist, showcasing early attempts at human-computer interaction.
- 1969: Shakey the Robot, developed by SRI International, became the first general-purpose mobile robot able to reason about its actions. Shakey's ability to navigate and interact with its environment marked a significant technological achievement.
- 1970s: Despite these advancements, the limitations of computing power and overly optimistic expectations led to the first "AI Winter." This period saw a reduction in funding and interest in AI research due to unmet expectations and the perceived failure of early AI projects.
1980s: Expert Systems and Renewed Interest
- 1980s: The development of expert systems, such as MYCIN, which was used for medical diagnoses, revived interest in AI. These systems emulated the decision-making abilities of human experts in specific fields, showcasing practical applications of AI technology.
- 1982: Japan's ambitious Fifth Generation Computer Systems project aimed to create supercomputers specifically designed for AI research, spurring global interest and competition in the field.
1990s: Machine Learning Emergence
- 1990s: AI research shifted towards machine learning, focusing on developing algorithms that could learn from data. This approach moved away from hardcoded rules towards models that could improve through experience.
- 1997: IBM’s Deep Blue made headlines by defeating world chess champion Garry Kasparov, demonstrating AI's potential in handling complex, strategic problem-solving tasks.
2000s: Big Data and Advanced Algorithms
- 2000s: The rise of big data and improvements in computing power enabled the development of more sophisticated AI algorithms. This period saw the growth of data-driven AI, leveraging vast amounts of information to train models.
- 2006: Geoffrey Hinton and his team introduced deep learning techniques, significantly improving AI capabilities. Deep learning, a subset of machine learning development, involves neural networks with many layers, allowing for more complex and accurate data processing.
2010s: AI in Everyday Life
- 2011: IBM's Watson won the quiz show Jeopardy!, showcasing AI’s ability to understand and process natural language at a high level.
- 2011-2014: The launch of AI-driven virtual assistants like Apple's Siri, Google Assistant, and Amazon's Alexa brought AI into everyday consumer technology, revolutionizing how people interacted with their devices.
- 2016: AlphaGo, developed by DeepMind, defeated a world champion Go player, marking a milestone in AI's ability to handle complex, strategic games with high levels of intuition and foresight.
- Late 2010s: AI's applications expanded across industries, including autonomous vehicles, healthcare diagnostics, and recommendation systems in e-commerce and entertainment. These applications showcased AI's versatility and transformative potential.
2020s: Integration and Ethical Focus
- 2020: Baidu's LinearFold AI algorithm accelerated COVID-19 vaccine development by predicting the virus's RNA sequence quickly.
- 2020: OpenAI released GPT-3, advancing natural language processing.
- 2021: OpenAI developed DALL-E, an image generation model from text prompts.
- 2022: The National Institute of Standards and Technology released its AI Risk Management Framework, and the White House introduced an AI Bill of Rights.
- 2022: OpenAI launched ChatGPT, rapidly gaining over 100 million users.
- 2023: Microsoft integrated AI into Bing, and Google announced Bard, later known as Gemini. OpenAI released GPT-4, enhancing language model capabilities.
- 2023: The Biden-Harris administration issued an executive order on AI safety and ethics, while Elon Musk's xAI launched the chatbot Grok.
- 2024: The European Union passed the Artificial Intelligence Act, ensuring safe and ethical AI use. Claude 3 Opus, developed by Anthropic, surpassed GPT-4 in performance.
Interested in learning more about how AI can benefit your business?
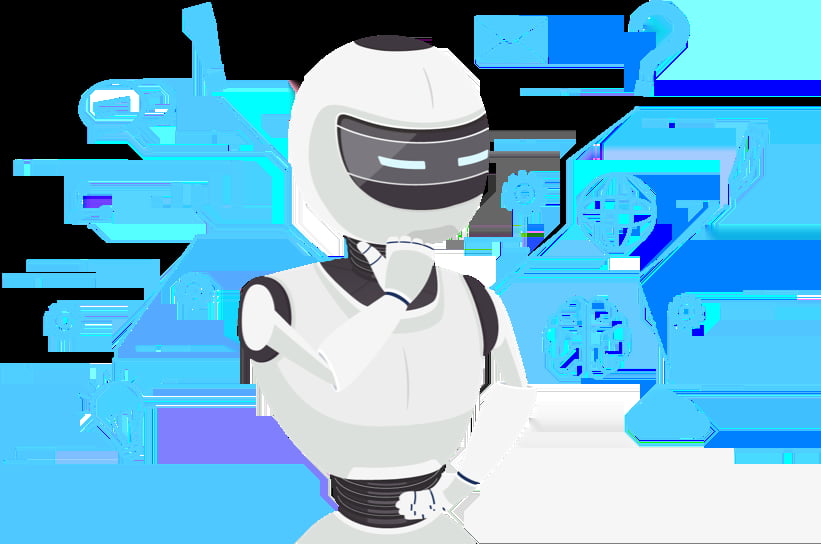
Understanding the Role of Artificial Intelligence
Artificial intelligence (AI) encompasses computer systems capable of performing tasks that typically require human intelligence, such as making predictions, identifying objects, interpreting speech, and generating natural language. These systems learn by processing vast amounts of data, detecting patterns, and modeling them to guide their decision-making. Often, humans oversee this learning process, reinforcing good decisions and correcting mistakes. Nevertheless, certain AI systems are built to learn independently without human oversight.
As these AI systems continue to learn, they become more proficient in their tasks, allowing them to adapt to new inputs and make decisions without explicit programming. Fundamentally, artificial intelligence seeks to enable machines to think and learn in a human-like manner, aiming to automate tasks and solve problems with greater efficiency.
What is the Significance of Artificial Intelligence?
Artificial intelligence (AI) aims to equip machines with human-like processing and analysis capabilities, making them a valuable ally in everyday life. AI can interpret and sort data on a massive scale, solve complex problems, and automate a variety of tasks simultaneously. This saves time and addresses operational gaps that humans might overlook.
AI is foundational to computer learning and finds applications in nearly every industry. In healthcare, finance, manufacturing, and education, AI aids in making data-driven decisions and handling repetitive or computationally intensive tasks efficiently.
Many existing technologies incorporate AI to enhance their capabilities. For instance, smartphones utilize AI assistants, e-commerce platforms employ recommendation systems, and vehicles boast autonomous driving features. Additionally, AI plays a crucial role in safeguarding people by powering fraud detection systems and operating robots in hazardous environments. It also spearheads advancements in healthcare research and climate initiatives.
In essence, AI is transforming how we interact with technology across various sectors, making processes more efficient, decisions more informed, and everyday life safer and more convenient.
How Does Artificial Intelligence Work?
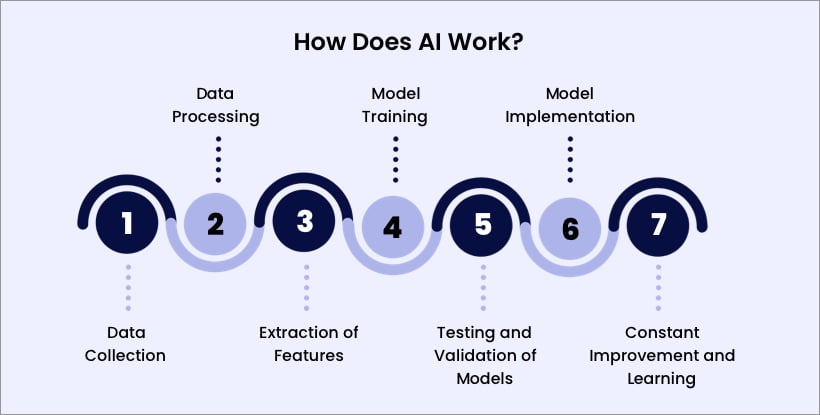
Though it may appear like an idea from the future, artificial intelligence (AI) is now pervasive in our daily lives. Artificial Intelligence (AI) operates in the background to improve human interactions with technology, from suggesting movies on streaming services to powering virtual assistants like Siri and Alexa. However, what is the real mechanism of AI operation?
Let's dissect the operation of AI systems in a way that makes sense while yet being thorough enough to recognize their complexity.
1. Data Collection
2. Data Processing
3. Extraction of Features
4. Model Training
5. Testing and Validation of Models
6. Model Implementation
7. Constant Improvement and Learning
Data collecting is the initial stage of every AI system. Data is necessary for AI to learn and make judgments. This information may be derived from many sources, including text, pictures, videos, and sensor data. An autonomous vehicle, for example, gathers information from its surroundings through the use of cameras, radar, and other sensors. AI systems in the healthcare industry may examine patient histories, photos, and medical information to find trends.
After it is gathered, the data must be cleaned and processed. Noise and irregularities are frequently present in raw data and need to be removed. Data processing entails addressing missing numbers, eliminating outliers, and arranging the data in an ordered way. This process makes sure the AI system receives high-quality data, which is essential for precise learning and prediction.
Feature extraction comes next after processing. Features are certain characteristics or qualities of the data that are relevant to the current task. Features in picture recognition, for instance, could be things like shapes, textures, and edges. Syntax, words, and phrases can all be considered features in natural language processing. The AI system can concentrate on the most crucial elements of the data by extracting pertinent attributes.
A model, developed by algorithms, is the central component of any AI system. By seeing patterns and connections in the data, the AI model gains knowledge throughout the training phase. In order to reduce errors, this entails feeding the model's processed data and changing its settings. Learning can be of two primary types:
The model's performance has to be verified and examined after training. This entails assessing the model's performance on unobserved data using a different set of data known as the validation and test datasets. By taking this step, you can make sure that the model is learning new inputs and not only remembering the ones from the training set.
The AI model is prepared for use after validation. The trained model is deployed when it is included in an actual application so that it can begin generating predictions or judgments. For instance, an e-commerce website may incorporate a trained artificial intelligence (AI) model for product recommendations to make suggestions to users based on their browsing history.
Artificial intelligence (AI) systems are dynamic; they constantly learn and advance. The system can update its model to maintain or enhance its performance when it is exposed to fresh data. "Model retraining" is the term for this continuous learning process, which is essential for adjusting to shifting data patterns and surroundings.
Different Types of Artificial Intelligence
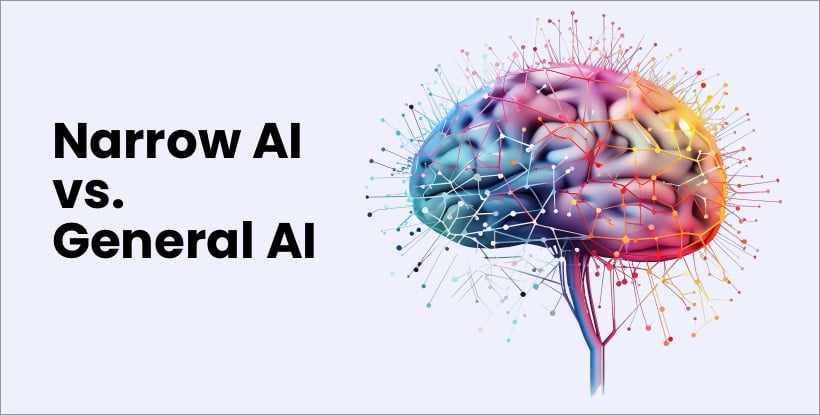
Artificial intelligence is a wide field that includes several forms and applications. Understanding the various types of AI and their numerous applications is critical, ranging from natural language processing to narrow AI.
Let us study the contrasts between these aspects to better comprehend how they interact to impact our businesses.
1. Narrow AI vs. General AI
2. Technical Overview of Machine Learning (ML)
- Supervised Learning: In supervised learning, the algorithm is trained on a labeled dataset, which means each input comes with an associated output. The model learns to predict the output from the input data. Common applications include spam detection in emails and image classification.
- Unsupervised Learning: Here, the algorithm is given data without explicit instructions on what to do with it. It must identify patterns and relationships within the data. Clustering and association are typical tasks for unsupervised learning, used in market basket analysis and customer segmentation.
- Reinforcement Learning: This approach involves training algorithms through a system of rewards and penalties. The AI system learns to achieve a goal in an uncertain, potentially complex environment. Reinforcement learning is widely used in robotics, gaming, and navigation systems.
3. Deep Learning and Neural Networks
4. Natural Language Processing (NLP) and Its Components
- Tokenization: Breaking down text into smaller units, such as words or phrases.
- Part-of-Speech Tagging: Identifying the grammatical parts of speech (nouns, verbs, adjectives, etc.) in a sentence.
- Named Entity Recognition (NER): Detecting and classifying proper nouns (names of people, organizations, locations).
- Sentiment Analysis: Determining the sentiment expressed in a piece of text, whether positive, negative, or neutral.
- Machine Translation: Translating text from one language to another using AI models.
5. Computer Vision Technologies
- Image Classification: Assigning a label to an entire image based on its content.
- Object Detection: Identifying and locating objects within an image.
- Image Segmentation: Partitioning an image into segments to simplify its analysis.
- Face Recognition: Identifying or verifying individuals based on facial features.
6. Robotics and AI
- Automation: Robots automate repetitive and dangerous tasks in industries like manufacturing, reducing the need for human intervention and increasing efficiency.
- Healthcare: Surgical robots perform precise operations, and service robots assist in elderly care, providing companionship and support.
- Exploration: AI-powered robots explore environments that are hazardous or inaccessible to humans, such as deep-sea exploration or space missions.
- Service Robots: These robots interact with customers in retail and hospitality, providing information, guiding customers, and even handling transactions.
AI is generally classified into two categories: Narrow AI and General AI.
Narrow AI, also known as Weak AI, is designed to perform specific tasks. It’s the type of AI we encounter most frequently today. Examples include virtual assistants like Siri and Alexa, recommendation systems on streaming platforms, and self-driving cars. These systems are incredibly good at their designated tasks but cannot perform functions outside their programmed parameters.
In contrast, General AI, or Strong AI, aims to replicate human intelligence's broad and adaptable nature. General AI can understand, learn, and apply knowledge across a wide range of tasks, much like a human can. While this concept fascinates scientists and engineers, it remains largely theoretical and is not yet a reality. The pursuit of General AI involves significant challenges, including replicating human consciousness and understanding complex, abstract concepts.
Machine Learning (ML) is a subset of AI focused on building systems that learn from data and improve over time without being explicitly programmed. The process of ML involves feeding algorithms large amounts of data and allowing them to learn patterns and make decisions based on that data.
ML algorithms fall into three main categories: supervised learning, unsupervised learning, and reinforcement learning.
Deep Learning is a specialized branch of ML that involves neural networks with many layers – hence the term “deep.” These neural networks are designed to mimic the human brain’s structure and function, consisting of interconnected nodes (neurons) organized into layers. Each layer processes input data and passes the results to the next layer, gradually refining the output.
Deep learning has revolutionized fields such as image and speech recognition, natural language processing, and autonomous driving. For example, convolutional neural networks (CNNs) are particularly effective in image recognition tasks, while recurrent neural networks (RNNs) excel in processing sequential data like speech and text.
The power of deep learning lies in its ability to automatically extract features from raw data, eliminating the need for manual feature engineering. However, deep learning models require large amounts of data and substantial computational resources to train effectively.
Natural Language Processing (NLP) enables machines to understand, interpret, and respond to human language in a way that is both meaningful and useful. NLP combines computational linguistics with machine learning and deep learning models to process human language
Key components of NLP include:
NLP powers various applications such as chatbots, virtual assistants, translation services, and sentiment analysis tools. With more natural and intuitive interactions between humans and machines.
Computer Vision is another crucial area of AI that enables machines to interpret and understand the visual world. By analyzing images and videos, computer vision systems can identify objects, track movements, and extract valuable information.
Key techniques in computer vision include:
Applications of computer vision are vast and varied. In healthcare, it assists in diagnosing diseases through medical imaging. In management and customer experiences. Autonomous vehicles rely heavily on computer vision to navigate and make real-time decisions.
Robotics, combined with AI, is pushing the boundaries of what machines can do. AI enables robots to perform tasks that require perception, decision-making, and adaptability. Robotics encompasses the design, construction, and operation of robots, while AI provides the intelligence that powers them.
Key areas where AI and robotics intersect include:
AI enhances the capabilities of robots by enabling them to learn from their environment, adapt to new situations, and improve their performance over time. This synergy between AI and robotics is leading to advancements across various sectors, driving innovation and improving productivity.
Core Technologies Behind AI
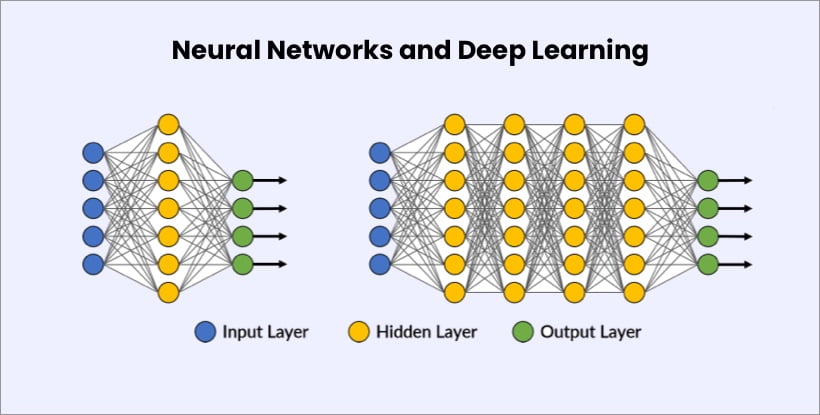
Artificial Intelligence (AI) has become a cornerstone of modern technology, driving advancements across various industries. But what makes AI tick? Let's explore the core technologies behind AI, delving into algorithms, neural networks, big data analytics, cloud computing, IoT integration, and advanced topics like Reinforcement Learning and Generative Adversarial Networks (GANs).
Algorithms and Data Structures
- Search Algorithms: These are used to explore problem spaces and find solutions. Examples include depth-first search (DFS) and breadth-first search (BFS).
- Optimization Algorithms: These algorithms, like gradient descent, find the best solution by minimizing or maximizing a function.
- Machine Learning Algorithms: These include supervised learning (e.g., decision trees, support vector machines), unsupervised learning (e.g., k-means clustering, principal component analysis), and reinforcement learning.
Neural Networks and Deep Learning Frameworks
- Feedforward Neural Networks: The simplest type, where data moves in one direction from input to output.
- Convolutional Neural Networks (CNNs): Used primarily in image and video recognition, CNNs are excellent at detecting patterns and features.
- Recurrent Neural Networks (RNNs): Designed for sequential data, RNNs are used in natural language processing and time series analysis.
- TensorFlow: Developed by Google, it's widely used for research and production.
- PyTorch: Preferred for its dynamic computation graph and ease of use, especially in research.
- Keras: An API that runs on top of TensorFlow, Keras simplifies building and training neural networks.
Big Data Analytics: Tools and Techniques
- Apache Hadoop: An open-source framework for distributed storage and processing of big data. Hadoop's HDFS (Hadoop Distributed File System) and MapReduce processing model are foundational components.
- Apache Spark: Known for its speed and ease of use, Spark processes large datasets in memory, making it ideal for machine learning tasks.
- Data Mining: Techniques like clustering, classification, and association are used to extract useful information from vast datasets.
Role of Cloud Computing in AI
- Scalability: Cloud platforms like AWS, Google Cloud, and Microsoft Azure offer scalable infrastructure, allowing AI models to process large datasets and perform complex computations.
- Accessibility: Cloud services provide access to powerful AI tools and frameworks without needing significant local hardware investment.
- Integration: Cloud platforms offer integrated AI services like machine learning, natural language processing, and computer vision APIs, enabling quick deployment of AI solutions.
Integration of the Internet of Things (IoT) with AI
- Data Collection: IoT devices generate vast amounts of data from sensors, cameras, and other sources.
- Data Processing: AI algorithms analyze IoT data to derive insights and make predictions.
- Actionable Insights: Based on the analysis, AI systems can trigger actions, such as adjusting a thermostat, sending alerts, or optimizing production processes.
Advanced Topics: Reinforcement Learning and Generative Adversarial Networks (GANs)
Reinforcement Learning (RL):
- Definition: RL is a type of machine learning where an agent learns to make decisions by performing actions and receiving feedback in the form of rewards or penalties.
- Applications: RL is used in robotics, gaming, and autonomous systems. For instance, Google's AlphaGo, which defeated human champions in the game of Go, leverages RL.
Generative Adversarial Networks (GANs):
- Definition: GANs consist of two neural networks, a generator, and a discriminator, that compete with each other. The generator creates fake data, while the discriminator evaluates its authenticity.
- Applications: GANs are used in image generation, style transfer, and data augmentation. They can create realistic images, enhance low-resolution images, and even generate art.
Data structures like arrays, linked lists, hash tables, and trees are vital for efficiently organizing and accessing data. For instance, decision trees use tree data structures to represent decisions and their possible consequences.
These frameworks provide pre-built functions and tools, accelerating the development of complex AI models.
Big data analytics helps AI models learn from extensive datasets, improving their accuracy and performance.
Cloud computing democratizes AI, making advanced technologies accessible to businesses and researchers worldwide.
For example, in smart cities, AI analyzes data from IoT sensors to manage traffic flow, reduce energy consumption, and enhance public safety. In manufacturing, AI-driven predictive maintenance uses IoT data to foresee equipment failures and schedule timely repairs.
These advanced techniques enable AI systems to learn and adapt in more sophisticated ways, opening new avenues for innovation.
AI's Transformative Impact on Business Operations
Artificial Intelligence (AI) is revolutionizing business operations across various industries, driving efficiency, productivity, and innovation. A report by McKinsey & Company reveals that AI has the potential to deliver an additional economic output of $13 trillion by 2030, boosting global GDP by approximately 1.2% annually.
One significant area where AI is making an impact is customer service. According to Gartner, by 2022, 70% of customer interactions were expected to involve emerging technologies such as machine learning applications, chatbots, and mobile messaging, up from 15% in 2018. This shift not only improves customer satisfaction but also reduces operational costs. IBM estimates that businesses can save up to 30% in customer support costs by implementing AI-driven chatbots.
In the realm of supply chain management, AI enhances predictive analytics and demand forecasting. A study by the Boston Consulting Group found that companies utilizing AI in their supply chains experienced a 15% reduction in logistics costs, a 35% reduction in inventory levels, and a 65% increase in service levels. These improvements lead to more efficient operations and significant cost savings.
Furthermore, AI's role in data analysis and decision-making cannot be overstated. AI algorithms analyze vast amounts of data to uncover patterns and insights that humans might miss. Forbes reports that 61% of businesses with an innovation strategy are using AI to identify opportunities in data that would otherwise be overlooked.
AI's transformative impact on business operations is undeniable. By automating routine tasks, enhancing customer interactions, optimizing supply chains, and providing deep data insights, AI empowers businesses to operate more efficiently and effectively. As AI technology continues to evolve, its influence on business operations will only grow, driving further advancements and opportunities.
Next, we will explore AI's profound impact on various industries, illustrating how different sectors are leveraging AI to revolutionize their practices and achieve unprecedented growth.
Ready to enhance your business efficiency with AI? Find out how AI is driving operational success across industries.
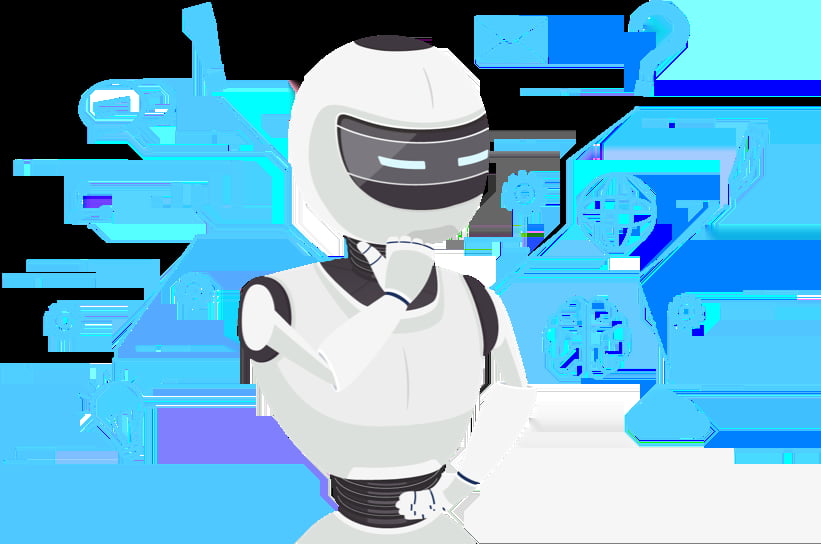
Practical Use-Cases of AI in Various Industries
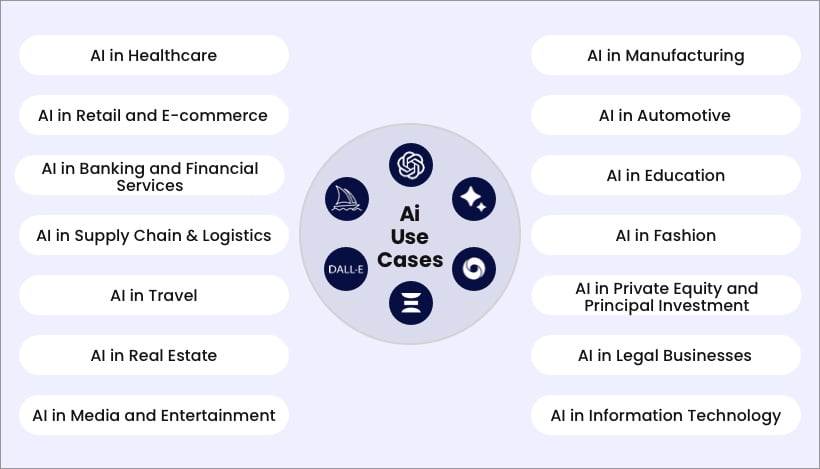
Artificial Intelligence (AI) is revolutionizing various industries by offering innovative solutions and optimizing operations. From enhancing customer experiences to automating complex processes, AI is proving to be a game-changer. Let's explore how AI is making a significant impact across different sectors.
AI in Healthcare
AI in Retail and E-commerce
AI in Fintech and Banking
AI in Music
AI in Logistics
AI in Travel
AI in Real Estate
AI in Media and Entertainment
AI in Manufacturing
AI in Automotive
AI in Education
AI in Fashion
AI in Fiction
AI in Movies
AI in Private Equity and Principal Investment
AI in Legal Businesses
AI in Information Technology
AI in Hospitality
AI is transforming healthcare with its ability to analyze vast amounts of data quickly and accurately. Medical imaging, for instance, is one area where AI excels. Algorithms can detect anomalies in X-rays, MRIs, and CT scans faster than human radiologists, leading to early diagnosis and treatment. Additionally, AI-powered chatbots and virtual assistants are providing patients with 24/7 support, answering queries, and even reminding them to take medications. This not only improves patient care but also reduces the burden on healthcare professionals.
In the retail and e-commerce sectors, AI is enhancing the shopping experience and optimizing operations. Personalized recommendations driven by AI analyze customer behavior and preferences, offering tailored product suggestions. Inventory management is also streamlined, as AI predicts demand, reducing overstock and stockouts. Furthermore, chatbots handle customer service inquiries, providing instant responses and freeing up human agents to tackle more complex issues.
AI in Fintech is reshaping banking and financial services by enhancing efficiency and security. Fraud detection systems powered by AI analyze transactions in real-time to prevent fraud. Robo-advisors offer personalized financial advice tailored to individual risk profiles and goals. Additionally, AI-driven algorithms optimize trading by analyzing market trends and executing precise decisions instantly.
AI is transforming the music industry by enhancing creativity and efficiency. Tools like Amper Music and AIVA compose original music, while machine learning algorithms curate personalized playlists on platforms like Spotify. AI also improves track mastering and sound quality. Additionally, AI-driven platforms like Jukedeck produce royalty-free music for videos and games, making music creation more accessible and innovative.
AI in logistics Supply Chain is transforming how goods are transported and managed. Predictive algorithms forecast demand, optimize delivery routes, and manage inventory, ensuring cost-effective and timely deliveries. AI-powered autonomous vehicles and drones enhance efficiency by streamlining transportation. Additionally, AI anticipates supply chain disruptions, enabling proactive measures to maintain smooth operations.
AI is revolutionizing the travel industry by personalizing the customer experience and streamlining operations. Chatbots and virtual assistants provide travelers with real-time information, from booking tickets to checking flight status. AI-powered recommendation engines suggest travel destinations, accommodations, and activities based on user preferences. Moreover, AI helps airlines optimize flight routes and manage schedules, reducing delays and enhancing fuel efficiency.
In real estate, AI simplifies property searches and transactions. AI-driven platforms analyze vast amounts of data, including property prices, neighborhood trends, and buyer preferences, to match potential buyers with suitable properties. Virtual tours powered by AI offer immersive experiences, allowing buyers to explore properties remotely. Additionally, AI assists in property management by predicting maintenance needs and optimizing energy usage.
The media and entertainment industry leverages AI to create personalized content and enhance viewer experiences. Streaming platforms like Netflix and Spotify use AI algorithms to recommend shows, movies, and music based on user preferences. AI also plays a significant role in content creation, with tools that generate music, write scripts, and even create visual effects. Additionally, AI-driven analytics help media companies understand audience behavior and preferences, enabling them to tailor their content strategy.
AI is driving efficiency and innovation in manufacturing. Predictive maintenance uses AI to monitor equipment performance and predict failures before they occur, reducing downtime and maintenance costs. Robotics powered by AI perform repetitive and hazardous tasks with precision, increasing productivity and safety. Additionally, AI optimizes supply chain management by predicting demand and adjusting production schedules accordingly.
The automotive industry is undergoing a transformation with the integration of AI. Autonomous vehicles, which rely heavily on AI, are set to revolutionize transportation by reducing accidents and improving traffic flow. AI also enhances driver assistance systems, such as adaptive cruise control and lane-keeping assistance, making driving safer and more comfortable. Furthermore, AI-powered predictive maintenance systems monitor vehicle health and alert drivers to potential issues, preventing breakdowns.
In education, AI personalises learning experiences and enhances administrative efficiency. AI-driven platforms adapt to individual learning styles and paces, providing personalized content and feedback. Virtual tutors and chatbots assist students with their queries, offering instant support. Additionally, AI automates administrative tasks such as grading and scheduling, allowing educators to focus more on teaching and student engagement.
The fashion industry is embracing AI to predict trends and optimize the supply chain. AI analyzes social media, runway shows, and sales data to forecast fashion trends, helping designers and retailers stay ahead of the curve. Virtual fitting rooms powered by AI allow customers to try on clothes virtually, enhancing the online shopping experience. Moreover, AI optimizes inventory management by predicting demand and reducing waste.
AI is changing the way fiction is written by helping writers come up with plots, characters, and conversations. For example, OpenAI's GPT-3 can write interesting short stories and come up with story ideas, which helps writers be more creative. AI can also look at trends and guess what will happen next, which helps writers write stories that people will want to read. Platforms that are powered by AI, like Reedsy and Scrivener, use AI to give you writing prompts and editing tips, which speed up the creative process.
AI is changing the movie business by making production better and producing more creative work. AI tools like ScriptBook look at scripts to see how well they might do at the box office, and companies like Cinelytic use AI to guess how well movies will do and make their marketing plans more effective. In visual effects (VFX), deep learning techniques are used to make animations and CGI that look real, like in "Avengers: Endgame."
AI is a valuable tool in private equity and principal investment, offering insights and optimizing decision-making processes. AI algorithms analyze financial data, market trends, and company performance to identify investment opportunities. Due diligence processes are also streamlined, as AI reviews documents and flags potential risks. Additionally, AI-driven predictive analytics help investors forecast returns and make informed decisions.
In the legal industry, AI automates routine tasks and enhances decision-making. AI-powered tools review and analyze legal documents, contracts, and case law, saving time and reducing errors. Predictive analytics assist lawyers in anticipating case outcomes and formulating strategies. Additionally, AI-driven chatbots provide clients with legal information and support, improving access to legal services.
The information technology sector leverages AI to enhance cybersecurity and optimize operations. AI-driven security systems detect and respond to cyber threats in real-time, protecting sensitive data. AI also automates IT support, with virtual assistants handling routine queries and troubleshooting. Moreover, AI optimizes software development processes by analyzing code, predicting issues, and suggesting improvements.
The hospitality industry benefits from AI by enhancing guest experiences and streamlining operations. AI-powered chatbots handle guest inquiries, from booking reservations to room service requests, providing instant responses. Personalization engines analyze guest preferences to offer tailored recommendations for dining, activities, and services. Additionally, AI optimizes energy management in hotels, reducing costs and environmental impact.
See AI in action! Explore real-world applications of AI in different industries and how it can benefit your business.
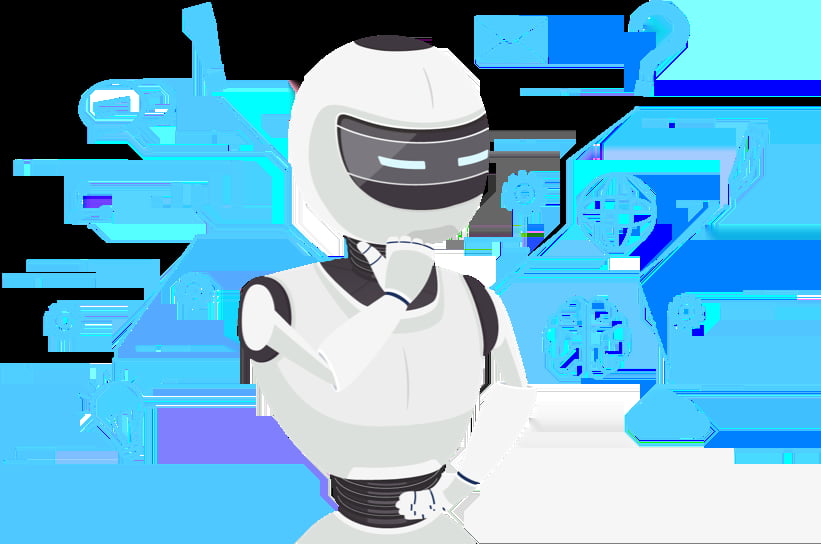
Benefits of AI for Businesses
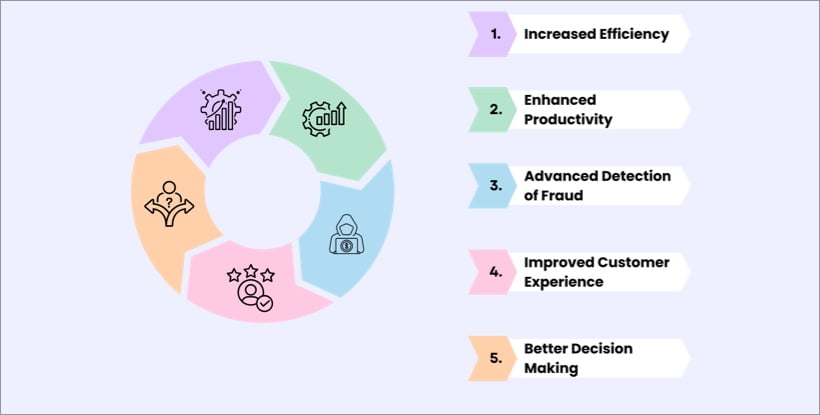
No longer confined to science fiction, AI is rapidly transforming the business landscape, offering a plethora of benefits that can empower companies to achieve new levels of efficiency, productivity, and profitability.
1. Increased efficiency
2. Enhanced productivity
3. Advanced Detection of Fraud
4. Improved customer experience
5. Better Decision Making
Efficiency is essential for corporate management. The more effectively a company runs, the more competitive it is in today's fast-paced market. This is where artificial intelligence comes into the picture.
One of the most promising characteristics of artificial intelligence in business is its capacity to streamline procedures and automate regular operations. For example, with AI-powered chatbots addressing customer support inquiries, human agents may concentrate on more difficult issues, resulting in a better customer experience.
Furthermore, AI-powered supply chain management solutions may streamline logistics, decreasing waste while saving time and resources. Meanwhile, AI-enabled financial systems can analyze massive volumes of data to uncover cost savings and assist businesses in making better-educated decisions.
AI systems can automate tasks that would normally be performed by employees. By taking over repetitive and time-consuming duties, these systems let staff focus on more strategic and value-added activities, resulting in higher production and a more efficient workforce.
Another area where artificial intelligence might improve productivity is project management. AI-powered project management solutions can improve workflows, identify bottlenecks, and deliver real-time updates on project status. This level of automation and data analysis can assist teams in completing tasks more quickly and efficiently, boosting productivity to new heights.
Considering this, AI-powered tools and systems can potentially increase productivity by lowering errors and boosting quality. For example, utilizing predictive analytics, AI-powered quality control systems may rapidly analyze production data and identify potential quality concerns before they become a problem. This saves time and ensures that the finished product satisfies the highest quality requirements.
Furthermore, AI can assist firms in optimizing their supply chain and logistics operations by analyzing massive volumes of data to discover inefficiencies, forecast demand, and optimize inventory management. This can lead to greater accuracy, shorter delivery times, and lower prices.
In contrast to traditional time-consuming fraud detection methods, AI can quickly process and analyze massive amounts of data. AI systems may detect possible fraud with high accuracy by evaluating patterns and trends in data from a variety of sources.
This is significantly more effective than traditional procedures, which rely on manual assessments and rule-based systems that expert fraudsters can quickly circumvent.
Also, AI has the potential to have a significant impact on identity verification. Machine learning algorithms can be used to evaluate biometric data and authenticate user identities, which can assist avoid identity fraud. This artificial intelligence technology significantly minimizes the risk of illegal access to sensitive information and financial damages.
On top of this, AI enhances fraud detection through behavioral analysis. AI systems can analyze customer behavior patterns, such as login attempts and transactions, to identify potential fraud. With machine learning algorithms, these systems can continuously adapt and improve their fraud detection capabilities.
In today's highly competitive industry, having an excellent customer experience is critical for retaining and recruiting new consumers. One of the most significant benefits of AI in this regard is its capacity to personalize client interactions.
AI systems can use client preferences and behavior data to generate tailored suggestions, offers, and experiences. This level of customization can result in higher client happiness, stronger loyalty, and, ultimately, better business outcomes.
Consider chatbots, for example. AI-powered chatbots can provide quick and efficient customer care, answering common inquiries around the clock and freeing up human agents to handle more difficult situations. They can also collect data on client interactions, which can provide useful insights into customer wants and preferences for future enhancements.
The marketing industry is another one where AI can improve the customer experience. In order to assist businesses develop more individualized and successful marketing efforts, artificial intelligence (AI) systems can analyze data to find new target markets. Through delivering an enhanced customer experience, enterprises may foster more robust customer relationships and stimulate expansion.
AI systems can handle and analyze massive amounts of data in real-time, giving businesses useful insights that can lead to continual efficiency gains. It is a game changer for firms who want to stay ahead of the curve.
In line with this, AI systems can spot trends, make forecasts, and provide important insights that can be used to inform decision-making by evaluating data from several sources. Unfortunately, this level of data analysis can only be performed by humans after a period.
Another area in which AI can help with decision-making is finance. AI-powered financial systems can analyze enormous volumes of data to uncover cost reductions, improve risk management, and assist businesses in making better investment decisions.
Challenges and Ethical Considerations in AI Integration
Artificial Intelligence (AI) has become a cornerstone of modern innovation, driving progress across various sectors from healthcare to finance. However, integrating AI into business operations and everyday applications is not without its challenges and ethical dilemmas. Understanding these complexities is crucial for organizations aiming to harness AI responsibly and effectively. Let's explore these challenges and ethical considerations in depth.
Technical Challenges: Data Quality, Algorithmic Bias, Scalability
- Data Quality: One of the most significant hurdles in AI integration is ensuring high-quality data. AI systems rely heavily on data to function accurately, and poor data quality can severely compromise their performance. Data must be clean, comprehensive, and relevant. Imagine training an AI model on outdated or incomplete datasets—such models are likely to produce inaccurate results, undermining the AI’s utility. Ensuring data quality involves rigorous preprocessing steps such as cleaning, normalization, and augmentation.
- Algorithmic Bias: Another technical challenge is algorithmic bias. AI systems learn from historical data, and if that data reflects existing biases, the AI will likely perpetuate these biases. For instance, an AI used in hiring processes might favor candidates from certain demographics if trained on biased data. Addressing algorithmic bias requires a multifaceted approach, including using diverse and representative datasets, applying fairness-aware algorithms, and conducting regular audits to detect and mitigate bias.
- Scalability: Scalability is also a critical concern. As AI models become more complex and data volumes increase, ensuring that these systems can scale efficiently is essential. This involves not only robust computational resources but also optimized algorithms that can handle large-scale data processing. Cloud computing platforms like AWS, Google Cloud, and Azure provide scalable infrastructure that can support AI applications, ensuring they remain efficient and effective as they grow.
Ethical Considerations in AI Development and Deployment
While technical challenges are significant, the ethical considerations in AI development and deployment are equally, if not more, important.
- Privacy and Security Issues in AI: AI systems often require vast amounts of data, which raises substantial privacy concerns. For example, AI-driven health apps collect sensitive patient information, which must be protected against breaches. Ensuring data privacy involves adhering to principles like data minimization—collecting only what is necessary—and implementing robust security measures such as encryption and secure access controls. Compliance with data protection regulations like GDPR and CCPA is also vital to ensure user privacy and trust.
- Regulatory and Compliance Requirements: As AI technologies evolve, regulatory frameworks are catching up to ensure these technologies are used ethically and responsibly. For instance, the European Union’s General Data Protection Regulation (GDPR) includes provisions for automated decision-making and profiling, mandating transparency and accountability. In the United States, the Federal Trade Commission (FTC) provides guidelines for the ethical use of AI in commercial practices. Organizations must stay informed about these regulations and ensure their AI systems comply with legal standards, which often includes maintaining transparency, accountability, and fairness.
Strategies for Ethical AI Development
Developing and deploying AI ethically requires a proactive approach. Here are several strategies that can help ensure AI systems are developed and used responsibly:
- Incorporating Ethical Guidelines: Organizations should establish clear ethical guidelines for AI development. These guidelines should cover aspects like fairness, transparency, accountability, and respect for user privacy. An AI ethics committee or advisory board can oversee the implementation of these guidelines and address ethical dilemmas as they arise.
- Transparent AI Systems: Transparency is key to building trust in AI systems. Developers should strive to create AI models that are interpretable and explainable. Explainable AI (XAI) techniques allow users to understand how decisions are made, which is particularly important in high-stakes areas like healthcare and finance. Providing users with clear explanations of how AI systems work and how decisions are made can help mitigate concerns about bias and fairness.
- Stakeholder Engagement: Engaging with a diverse range of stakeholders, including users, industry experts, and ethicists, can provide valuable insights into the ethical implications of AI systems. Regular consultations and feedback loops can help identify potential ethical issues early in the development process. Involving stakeholders in the design and testing phases can also ensure that AI systems are aligned with societal values and expectations.
- Continuous Monitoring and Evaluation: Ethical AI development is an ongoing process that requires continuous monitoring and evaluation. Implementing robust monitoring systems can help detect and address issues such as bias, discrimination, and unintended consequences. Regular audits and assessments can ensure that AI systems remain aligned with ethical guidelines and regulatory requirements.
Promoting Diversity in AI Teams: Diverse development teams are more likely to consider a broader range of ethical implications and potential biases. Promoting diversity in AI teams can help ensure that different perspectives are considered during the development process. Encouraging inclusive hiring practices and fostering a culture of diversity and inclusion can contribute to more ethical AI development.
Education and Training: Educating developers, data scientists, and business leaders about the ethical implications of AI is crucial. Providing training on topics like bias mitigation, data privacy, and regulatory compliance can help ensure that ethical considerations are integrated into the AI development process. Organizations should also encourage ongoing learning and professional development to stay current with evolving ethical standards and best practices.
Collaboration and Industry Standards: Collaboration between industry, academia, and government is essential to developing and implementing ethical AI standards. Establishing industry-wide standards and best practices can help ensure consistency and accountability in AI development. Organizations should actively participate in industry forums and initiatives focused on ethical AI to stay informed and contribute to the development of ethical guidelines.
The AI Development Process: From Concept to Deployment
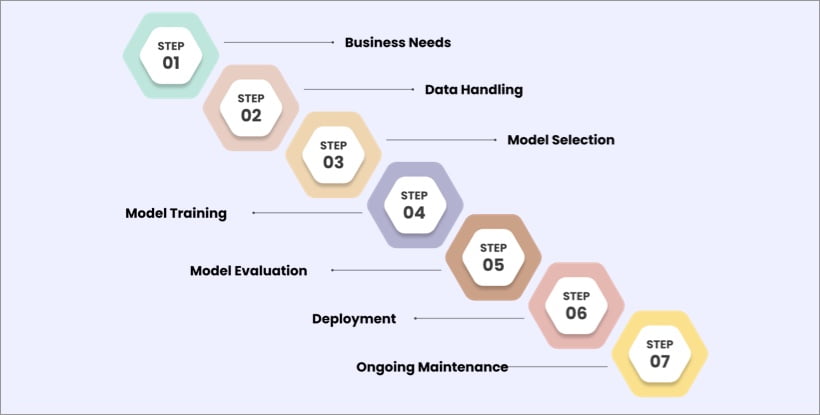
In the fast-evolving world of technology, artificial intelligence (AI) stands out as a transformative force, reshaping industries and creating new opportunities. However, developing AI solutions is a complex process that requires a systematic approach. Let’s dive into the AI development process, covering each essential step from identifying business needs to ongoing maintenance and model retraining.
Identifying Business Needs and Defining AI Use Cases
- What specific business challenges are you facing?
- What goals are you aiming to achieve with AI?
- How do you envision AI providing a competitive edge in your industry?
Data Collection, Preprocessing, and Augmentation
- What types of data do you currently collect?
- How is your data stored and managed?
- Are there any data quality issues we need to address?
Technical Considerations in Choosing AI Models and Algorithms
- What is the nature of the problem you want to solve (e.g., classification, regression, clustering)?
- What type of data are we working with (e.g., structured, unstructured, text, images)?
- What are your performance requirements (e.g., accuracy, speed, interpretability)?
Model Training: Techniques, Tools, and Best Practices
- Train-test split: Dividing data into training and testing sets to evaluate performance.
- Cross-validation: Using techniques like k-fold cross-validation to ensure generalization.
- Hyperparameter tuning: Optimizing hyperparameters to enhance performance.
- Regularization: Applying techniques like L1, L2, and dropout to prevent overfitting.
Evaluation Metrics and Validation Methods
- Accuracy: How well does the model predict correctly?
- Precision and Recall: How relevant are the positive predictions?
- F1 Score: A balance between precision and recall.
- Mean Squared Error (MSE): Average squared difference between predicted and actual values for regression tasks.
Deployment Strategies and Integration with Existing Systems
- What are your deployment preferences (e.g., on-premises, cloud, edge)?
- How do you plan to integrate the AI solution with your current infrastructure?
- What scalability requirements do you have?
Ongoing Monitoring, Maintenance, and Model Retraining
- What key performance metrics should we track?
- How will we address anomalies and performance issues?
- What is your plan for model retraining to maintain performance?
As an AI development company, we begin by engaging with our clients to thoroughly understand their business needs and objectives. We ask key questions to pinpoint areas where AI can add significant value
By addressing these questions, we can identify clear AI use cases tailored to your business. For example, if you’re in retail, we might explore AI applications in customer personalization, inventory management, and sales forecasting. Defining these use cases helps set clear objectives and ensures alignment with your business goals.
Data is the backbone of AI. To build robust AI solutions, we ask our clients:
With this information, we gather relevant data from various sources, ensuring it’s comprehensive and representative. The data is then preprocessed to remove noise, normalize formats, and handle missing values, ensuring high-quality input for our models. If data is limited, we employ data augmentation techniques to create a more robust dataset, enhancing the model's performance.
Choosing the right AI models and algorithms is critical. We collaborate with our clients to understand:
With this information, we gather relevant data from various sources, ensuring it’s comprehensive and representative. The data is then preprocessed to remove noise, normalize formats, and handle missing values, ensuring high-quality input for our models. If data is limited, we employ data augmentation techniques to create a more robust dataset, enhancing the model's performance.
Model training is where the AI solution starts to take shape. We follow best practices to ensure robust model performance:
We utilize tools such as TensorFlow, PyTorch, and Scikit-learn for efficient model training and validation, ensuring our models meet the highest standards of accuracy and reliability.
Evaluating AI model performance is crucial. We work with our clients to identify the most relevant evaluation metrics:
We use validation methods like cross-validation, hold-out validation, and bootstrap validation to ensure our models perform well on unseen data, identifying any issues like overfitting or underfitting early in the process.
Deploying AI solutions involves seamless integration with existing systems. We ask our clients:
Based on these discussions, we develop APIs and microservices to enable smooth communication between the AI solution and other applications. We ensure compatibility and interoperability to facilitate a seamless deployment process.
AI development doesn’t end with deployment. Continuous monitoring and maintenance are essential. We collaborate with our clients to establish:
We set up monitoring tools like Prometheus, Grafana, and ELK Stack for real-time performance tracking and alerting. Regular model retraining is scheduled to update training data, ensuring the AI solution remains effective and reliable.
Debut Infotech, a leading expert in artificial intelligence consulting and development, expertly navigates the entire generative AI development lifecycle. The process starts with a comprehensive assessment of project requirements, ensuring a thorough understanding of stakeholder expectations. Our strategic approach includes model selection, training methodologies, and resource allocation, followed by careful design, development, rigorous testing, and continuous optimization. The final stages involve seamless integration into the client's ecosystem, backed by ongoing support to adapt to evolving needs. Our specialized team excels in providing strategic guidance for AI implementation, addressing unique challenges, and creating tailored solutions to enhance operational efficiency.
Leading AI Technology Trends to Follow
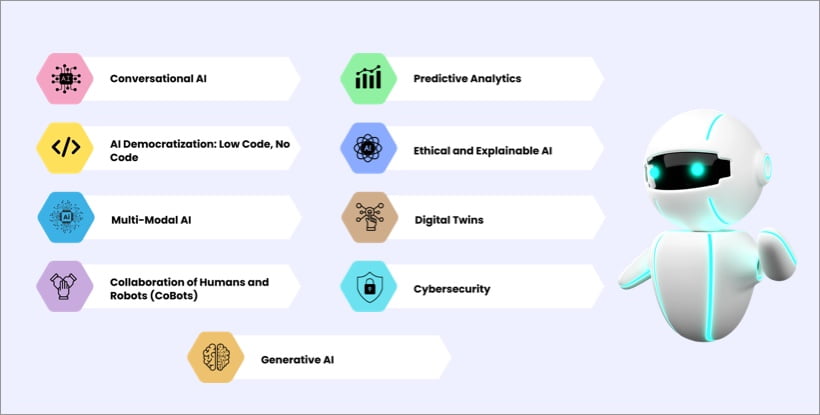
AI is advancing at an extraordinary pace, reshaping the business landscape significantly. Entrepreneurs must stay informed about current AI trends to maintain a competitive edge. Let's delve into the top AI trends of 2024.
Conversational AI
Predictive Analytics
AI Democratization: Low Code, No Code
Ethical and Explainable AI
Multi-Modal AI
Digital Twins
Collaboration of Humans and Robots (CoBots)
Cybersecurity
Key AI Applications in Cybersecurity:
- Facial Recognition: Dominant in biometric authentication, it helps prevent unauthorized access.
- Cyber Threat Hunting: Proactively detects and responds to cyber threats, reducing the risk of attacks.
- Surveillance: AI-powered surveillance provides 24/7 support, detecting suspicious behavior and alerting authorities.
Generative AI
ChatGPT
DALL-E
Conversational AI applications, such as chatbots, automate complex, repetitive, and rule-based tasks, enhancing customer experiences and improving productivity. According to Statista, the chatbot market is estimated to reach around $1.25 billion by 2025. The use of advanced conversational AI across industries is expected to rise, with integrated AI enhancing product efficiency and handling complex queries.
Predictive analytics enables businesses to make informed decisions, optimizing inventory, improving delivery times, reducing operational costs, and increasing sales and revenues. In manufacturing, predictive maintenance algorithms predict unexpected machine failures, preventing costly breakdowns. With reduced sensor costs, edge computing, and edge AI, predictive maintenance is now more accessible.
Low-code and no-code AI platforms allow businesses to customize intelligent systems through drag-and-drop methods and pre-built templates. This trend automates repetitive tasks and programs AI tools for data analysis. By 2025, it's expected that 70% of new web and software applications will utilize low-code or no-code platforms.
As AI models become more sophisticated, their lack of transparency can lead to discrimination and misuse. Explainable AI addresses this by examining patterns in AI models and clarifying decision-making processes. This fosters trust and transparency, enabling businesses to interpret and communicate AI outcomes effectively.
Multi-modal AI integrates multiple data modalities (speech, images, video, audio, text, etc.) to create a holistic cognitive experience. Enterprises use multi-modal AI to enhance natural language understanding, visual perception, and voice recognition. For instance, Google DeepMind's Gato performs language, visual, and robotic tasks.
Digital twins create virtual replicas of physical assets, processes, or systems, allowing real-time monitoring, analysis, and optimization. This trend is popular in Industry 4.0 and IoT, providing innovative ways to predict real-world scenarios like disease progression and economic impacts. NVIDIA and Siemens have partnered to create an industrial metaverse using digital twin technology.
Collaborative Robots (CoBots) enhance productivity, safety, and efficiency by working alongside humans in industries. AI-powered CoBots exemplify the synergy between human intelligence and robotic precision, taking over intricate tasks in manufacturing and enabling humans to focus on design, programming, and maintenance.
AI-based cybersecurity is crucial for detecting vulnerabilities and preventing cyber threats. The AI cybersecurity market is expected to reach $60.6 billion by 2028, with a CAGR of 21.9% from 2023 to 2028. AI enhances defense mechanisms, ensuring data security and uninterrupted operations.
Generative AI Development, a subset of machine learning, creates new data, content, and 3D/2D images using existing datasets. In healthcare, it aids in rendering prosthetic limbs, designing organic molecules, and diagnosing diseases early. Notable examples include:
One of the most prominent examples of cutting-edge natural language processing technology is ChatGPT, which was developed by OpenAI. Using techniques from deep learning, ChatGPT has exhibited impressive capabilities in generating language that is eerily similar to that of humans. This has resulted in major improvements to customer service, content creation, and instructional applications.
Recent studies have demonstrated that chatbots powered by artificial intelligence, such as ChatGPT, are capable of handling up to 80 percent of regular customer inquiries, thereby significantly decreasing response times and operational expenses. ChatGPT developers are revolutionizing various sectors by automating complex communication activities and enhancing user engagement. It is able to comprehend and generate text with contextual correctness, which is a significant advantage.
DALL-E, another innovation from OpenAI, excels in creating highly realistic and imaginative images from textual descriptions. This model leverages the power of deep learning and generative adversarial networks (GANs) to produce visuals that are not only accurate but also creatively rich.
In the domain of advertising and design, DALL-E is revolutionizing content creation by enabling designers to generate custom images quickly and efficiently. According to a recent survey, companies using AI-driven image generation tools like DALL-E have reported a 30% increase in design productivity and a 20% reduction in project turnaround times. This capability allows for unprecedented creativity and personalization in visual content.
The Rising Future of Artificial Intelligence
With the rapid advancements in AI technologies, the impact of AI across industries is becoming increasingly profound. As these technologies continue to progress, we anticipate the emergence of smarter and more intuitive systems, intelligent automation, personalized experiences, and enhanced decision-making capabilities across various sectors.
The latest AI and ML trends, such as explainable AI, collaborative robots (cobots), generative AI, conversational AI, computer vision, and the democratization of AI, are poised to address complex challenges effectively. From revolutionizing healthcare diagnostics to enhancing financial fraud detection, these innovations are set to transform industries.
Leveraging these cutting-edge AI trends, businesses worldwide are finding novel ways to integrate artificial intelligence, setting new benchmarks for innovation and excellence. This integration paves the way for unprecedented advancements and the creation of superior, AI-driven solutions.
Ultimately, AI is steering us toward a future where humans and robots collaborate seamlessly, ushering in a new era of innovation and limitless possibilities. The promise of AI lies in its potential to reshape how we live and work, offering advancements that will revolutionize our daily lives and professional landscapes.
Given the swift progress in artificial intelligence, now is the ideal time to integrate AI into your business operations. To do this successfully, you need to collaborate with a top-tier AI development company that boasts a team of highly skilled developers. Henceforth, we will walk you through the process of hiring AI developers to seamlessly embed AI into your business and propel your success forward.
How Do You Hire AI Developers for Your Next Project?
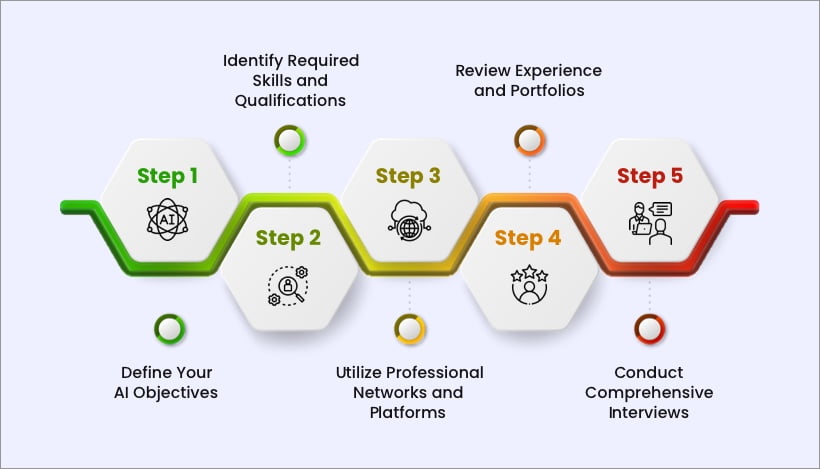
The integration of Artificial Intelligence (AI) into business operations is no longer a luxury but a necessity. Companies across various industries are leveraging AI to improve efficiency, enhance customer experiences, and drive innovation. However, successfully incorporating AI requires more than just technology; it demands a team of skilled AI developers who can tailor solutions to meet your specific business needs. Here’s a comprehensive guide on how to hire AI developers for your next project.
Step 1: Define Your AI Objectives
Step 2: Identify Required Skills and Qualifications
- Programming Proficiency: Expertise in languages such as Python, R, and Java.
- AI Frameworks Experience with TensorFlow, PyTorch, Keras, etc.
- Machine Learning In-depth knowledge of algorithms, neural networks, and deep learning.
- Data Handling Skills in data preprocessing, mining, and database management.
- Problem-Solving Ability to devise solutions for complex business problems.
- Soft Skills Effective communication, teamwork, and the capacity to simplify technical concepts.
Step 3: Utilize Professional Networks and Platforms
- Professional Networks Platforms like LinkedIn are invaluable for connecting with AI professionals.
- Online Communities: Engage with communities on GitHub, Stack Overflow, and Kaggle.
- Events: Attend AI conferences, workshops, and meetups to network with experts and stay abreast of industry trends.
Step 4: Review Experience and Portfolios
Step 5: Conduct Comprehensive Interviews
- Technical Assessments: Include coding challenges and problem-solving exercises relevant to the job.
- Project Discussions: Have candidates explain previous projects, challenges faced, and solutions devised.
- Cultural Fit: Determine if they align with your team’s dynamics and company values.
Before you begin the hiring process, it’s essential to clearly outline your AI goals. Are you aiming to enhance customer service through chatbots, improve data analytics, automate processes, or develop new AI-driven products? Having a clear understanding of your objectives will help you identify the right candidates who possess the specific skills and experience needed for your project.
AI development requires a blend of technical and analytical skills. Key qualifications to look for include:
Finding top AI talent often requires a strategic approach:
Evaluate candidates based on their practical experience and past projects. Request portfolios showcasing their work, including AI models, research papers, or open-source contributions. This helps you gauge their capabilities and application of skills in real-world scenarios.
Ensure the interview process is thorough, assessing both technical skills and cultural fit:
Looking to integrate AI into your business? Partner with our expert AI developers and take your operations to the next level.
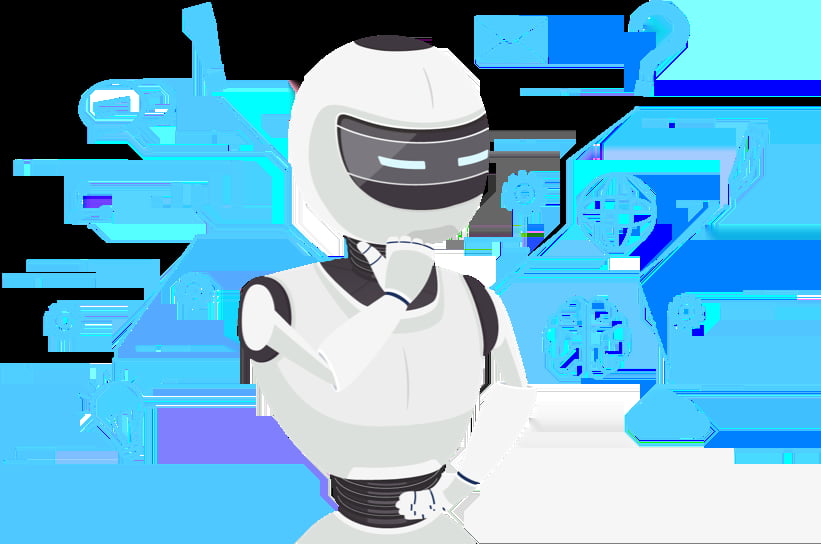
Partner with Us to Enterprise AI in Your Business Operations
At Debut Infotech, we are dedicated to transforming the way businesses operate by leveraging cutting-edge technology. Founded with a vision to harness the power of AI and other emerging technologies, we have grown into a premier AI development company. Having a team of 100+ seasoned AI & Generative AI engineers who are passionate about innovation and committed to delivering exceptional results. With a focus on quality, we provide tailored solutions that drive efficiency, enhance customer experiences, and foster growth. We partner with businesses across various industries to help them navigate the complexities of digital transformation and stay ahead in a competitive market.
Generative AI Solutions
Smart AI Assistants and Chatbots
Innovative AI Product Development
Recommendation Engines
AI-as-a-Service (AIaaS) Solutions
Cutting-Edge Automation Solutions
AIOps Solutions
MLOps Solutions
Bespoke Development of Enterprise AI Solutions
Image Recognition and Analysis
Our Capabilities, Team, and Success Rate
With extensive expertise, our team of Generative AI consultants and developers specializes in Generative AI consulting, development, and integration services. We craft advanced Generative AI solutions using cutting-edge technologies like GPT-4, GPT-3, GPT-3.5, Midjourney, and DALL-E. Our AI models excel in visual content analysis, enabling brand identification, data analysis, business intelligence, visualization, and more.
Within our suite of AI services, we excel in seamless AI chatbot development, easily integrable across various platforms, websites, and applications. As a leading AI services provider, we design bespoke conversational bots to facilitate efficient client interactions and elevate overall customer experiences.
Our bespoke AI development services fuel innovation, enhancing operational efficiency with a focus on scalability and reliability. Whether developing recommendation engines, natural language processing applications, or robust predictive analysis tools, our adept developers engineer user-friendly solutions that seamlessly integrate with your existing systems.
Explore the extensive benefits of our tailored recommendation engine development services. Elevate customer satisfaction and retention while boosting revenue streams through precise recommendations that drive conversions. Experience unparalleled efficiency as we streamline your business processes.
Empower your AI capabilities with our AIaaS solutions, enabling scalable AI infrastructure without the need for significant investments in costly hardware and specialized AI talent. Overcome the complexities and expenses associated with in-house AI solution development while reaping the full benefits of seamless AI implementation.
Specializing in the deployment and integration of technology-driven processes and systems, our team of AI experts utilizes advanced technologies such as Robotic Process Automation (RPA) to enhance business accuracy and expedite turnaround times. Through the automation of repetitive tasks, operational streamlining, and efficiency improvement, we empower businesses across diverse industries to optimize their operations.
As a committed AI development company, our AIOps solutions enable organizations to proactively ensure continuous application performance, resulting in exceptional customer experiences. Our services swiftly reduce IT costs, enabling businesses to optimize operations and enhance their bottom line.
We offer comprehensive MLOps servicess that streamline the ML lifecycle from development to deployment. Our automation reduces manual intervention, while robust CI/CD pipelines ensure seamless model validation and deployment. With scalable infrastructure management, we support efficient handling of large datasets and complex models, ensuring reliability and scalability.
Our AI experts meticulously design and implement custom solutions tailored to meet the unique demands of your enterprise. Whether optimizing supply chains or elevating customer experiences, our enterprise AI solutions are crafted to amplify operational efficiency and foster innovation within your specific industry.
Harness the power of our image recognition and analysis solutions to extract valuable insights from visual data. Our advanced AI algorithms are adept at identifying objects, faces, patterns, and more, enabling businesses to streamline processes, improve decision-making, and enhance customer engagement. Experience the transformative impact of image recognition technology in unlocking new opportunities and driving innovation across various industries.
Our strength lies in our team of highly skilled professionals, including data scientists, AI engineers, and domain experts. With over 100 specialists, we bring a wealth of knowledge and experience to every project. Our collaborative approach ensures that we stay at the forefront of AI advancements and can tackle even the most complex challenges.
We as a leading AI consulting company take pride in our impressive success rate, having delivered over 100 AI-enabled solutions to satisfied clients across various industries. Our projects have led to significant improvements in efficiency, cost reduction, and customer satisfaction. For example, our AI-driven chatbots have reduced operational costs by up to 30%, while our predictive analytics tools have increased decision-making accuracy by 95%.
We have helped numerous businesses customize AI-enabled solutions to meet their unique requirements. By understanding each client's specific challenges and goals, we develop tailored solutions that drive measurable outcomes. Our commitment to excellence and innovation has earned us a reputation as a trusted partner in AI development and integration.
Essential AI Development Glossary of Terms to Understand
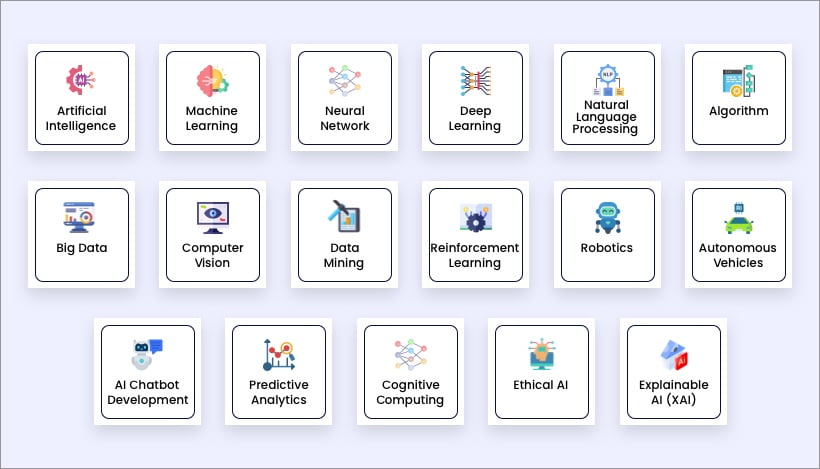
Understanding the key terms related to Artificial Intelligence (AI) is essential for navigating this complex field. Below, we provide detailed explanations of important AI concepts and terminology.
Artificial Intelligence (AI)
Machine Learning (ML)
Neural Network
Deep Learning
Natural Language Processing (NLP)
Algorithm
Big Data
Computer Vision
Data Mining
Reinforcement Learning
Robotics
Autonomous Vehicles
AI Chatbot Development
Predictive Analytics
Cognitive Computing
Ethical AI
Explainable AI (XAI)
AI refers to the creation of systems or machines that can perform tasks requiring human intelligence. These tasks include problem-solving, learning, language understanding, and pattern recognition. AI can be broadly categorized into narrow AI (designed for specific tasks) and general AI (with cognitive abilities similar to humans).
A subset of AI, machine learning development involves developing algorithms that allow computers to learn from data and improve their performance over time without being explicitly programmed. Key techniques include supervised learning, unsupervised learning, and reinforcement learning.
Neural networks are a series of algorithms that attempt to recognize underlying relationships in data through a process that mimics the human brain. They are composed of layers of nodes, with each node representing a neuron. Neural networks are the foundation for deep learning and are used in various AI applications, including image and speech recognition.
A branch of machine learning, deep learning uses neural networks with many layers (hence the term "deep"). These deep neural networks can model complex patterns in large amounts of data, making them powerful tools for tasks such as natural language processing, image analysis, and more.
NLP is a field of AI focused on the interaction between computers and humans through natural language. It encompasses tasks like speech recognition, language translation, sentiment analysis, and text generation. NLP allows machines to understand, interpret, and respond to human language meaningfully.
An algorithm is a set of rules or steps designed to solve a problem or perform a task. In AI, algorithms process data and make decisions or predictions based on that data. Machine learning and deep learning rely heavily on sophisticated algorithms to function.
Big data refers to extremely large datasets that can be analyzed computationally to reveal patterns, trends, and associations. In AI, big data is crucial because it provides the raw material needed to train machine learning models. The ability to process and analyze big data has been a key driver of AI advancements.
Computer vision is a field of AI that enables machines to interpret and make decisions based on visual data from the world. This includes tasks like image and video recognition, object detection, and facial recognition. Computer vision is used in various applications, from autonomous vehicles to medical imaging.
Data mining involves examining large datasets to discover patterns, correlations, and insights. This process is critical in AI for extracting useful information from raw data. Data mining techniques are used in various applications, including market analysis, fraud detection, and scientific research.
Reinforcement learning is a type of machine learning where an agent learns to make decisions by performing actions and receiving feedback in the form of rewards or penalties. This trial-and-error approach helps the agent learn the optimal behavior for achieving its goals. It is widely used in robotics, game playing, and autonomous systems.
Robotics is an interdisciplinary branch of AI that integrates computer science and engineering to design, construct, and operate robots. Robots can perform a wide range of tasks, from simple repetitive actions to complex decision-making and autonomous navigation. AI enhances robots' capabilities, making them more adaptable and intelligent.
Autonomous vehicles, or self-driving cars, use AI to navigate and operate without human intervention. These vehicles rely on a combination of sensors, cameras, radar, and AI algorithms to understand their environment and make driving decisions. Autonomous vehicles have the potential to revolutionize transportation by improving safety and efficiency.
AI chatbot development is a software application designed to simulate conversation with human users. Chatbots are commonly used in customer service to handle inquiries and provide assistance. They can operate through text or voice interactions and use NLP to understand and respond to user inputs.
Predictive analytics involves using statistical techniques and machine learning algorithms to analyze historical data and make predictions about future events. Businesses use predictive analytics to forecast trends, identify risks, and make informed decisions. It is applied in fields like finance, marketing, healthcare, and supply chain management.
Cognitive computing aims to create systems that mimic human thought processes. These systems use AI, machine learning, NLP, and other technologies to understand, reason, and interact naturally with humans. Cognitive computing applications include virtual assistants, decision support systems, and personalized recommendations.
Ethical AI refers to the development and deployment of AI systems in a manner that is fair, transparent, and accountable. Ethical considerations in AI include addressing biases in data, ensuring privacy, preventing discrimination, and maintaining human oversight. The goal is to create AI technologies that benefit society while minimizing harm.
Explainable AI focuses on creating AI models whose decisions and actions can be understood by humans. This is crucial for building trust and ensuring that AI systems are used responsibly. Techniques for explainable AI include using simpler models, visualizing model decisions, and providing clear explanations for predictions.